Full Paper View Go Back
Mansoor Farooq1 , Mubashir Hassan Khan2
Section:Research Paper, Product Type: Journal
Vol.12 ,
Issue.1 , pp.1-8, Feb-2024
Online published on Feb 28, 2024
Copyright © Mansoor Farooq, Mubashir Hassan Khan . This is an open access article distributed under the Creative Commons Attribution License, which permits unrestricted use, distribution, and reproduction in any medium, provided the original work is properly cited.
View this paper at Google Scholar | DPI Digital Library
How to Cite this Paper
- IEEE Citation
- MLA Citation
- APA Citation
- BibTex Citation
- RIS Citation
IEEE Style Citation: Mansoor Farooq, Mubashir Hassan Khan, “EDeLeaR: Edge-based Deep Learning with Resource Awareness for Efficient Model Training and Inference for IoT and Edge Devices,” International Journal of Scientific Research in Network Security and Communication, Vol.12, Issue.1, pp.1-8, 2024.
MLA Style Citation: Mansoor Farooq, Mubashir Hassan Khan "EDeLeaR: Edge-based Deep Learning with Resource Awareness for Efficient Model Training and Inference for IoT and Edge Devices." International Journal of Scientific Research in Network Security and Communication 12.1 (2024): 1-8.
APA Style Citation: Mansoor Farooq, Mubashir Hassan Khan, (2024). EDeLeaR: Edge-based Deep Learning with Resource Awareness for Efficient Model Training and Inference for IoT and Edge Devices. International Journal of Scientific Research in Network Security and Communication, 12(1), 1-8.
BibTex Style Citation:
@article{Farooq_2024,
author = {Mansoor Farooq, Mubashir Hassan Khan},
title = {EDeLeaR: Edge-based Deep Learning with Resource Awareness for Efficient Model Training and Inference for IoT and Edge Devices},
journal = {International Journal of Scientific Research in Network Security and Communication},
issue_date = {2 2024},
volume = {12},
Issue = {1},
month = {2},
year = {2024},
issn = {2347-2693},
pages = {1-8},
url = {https://www.isroset.org/journal/IJSRNSC/full_paper_view.php?paper_id=440},
publisher = {IJCSE, Indore, INDIA},
}
RIS Style Citation:
TY - JOUR
UR - https://www.isroset.org/journal/IJSRNSC/full_paper_view.php?paper_id=440
TI - EDeLeaR: Edge-based Deep Learning with Resource Awareness for Efficient Model Training and Inference for IoT and Edge Devices
T2 - International Journal of Scientific Research in Network Security and Communication
AU - Mansoor Farooq, Mubashir Hassan Khan
PY - 2024
DA - 2024/02/28
PB - IJCSE, Indore, INDIA
SP - 1-8
IS - 1
VL - 12
SN - 2347-2693
ER -
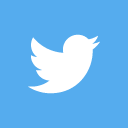
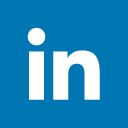
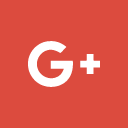
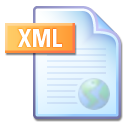
Abstract :
Deep learning has emerged as a powerful technique for processing and extracting insights from complex data. However, the resource-constrained nature of edge devices poses significant challenges to the deployment of deep learning models at the network edge. This research proposes a novel algorithm called EDeLeaR, which stands for Edge-based Deep Learning with Resource-awareness, to enable efficient model training and inference in edge computing environments. EDeLeaR leverages adaptive resource allocation and optimization techniques to maximize the utilization of limited computational resources while preserving model accuracy and minimizing latency. This paper presents the design, implementation, and evaluation of EDeLeaR, showcasing its effectiveness through comprehensive experiments on real-world edge devices.
Key-Words / Index Term :
Edge Computing, Deep learning, IoT, Network Security, Resource-awareness, Model Compression & Collaborative learning
References :
[1] F. Wang, M. Zhang, X. Wang, X. Ma and J. Liu, "Deep Learning for Edge Computing Applications: A State-of-the-Art Survey," IEEE Access, Vol.8, pp.58322-58336, 2020. doi: 10.1109/ACCESS.2020.2982411.
[2] Guillén, M.A., Llanes, A., Imbernón, B. et al. Performance evaluation of edge-computing platforms for the prediction of low temperatures in agriculture using deep learning. Journal of Supercomputing 77, pp.818–840, 2021. https://doi.org/10.1007/s11227-020-03288-w
[3] G. Muhammad and M. S. Hossain, "Emotion Recognition for Cognitive Edge Computing Using Deep Learning," in IEEE Internet of Things Journal, Vol.8, No.23, pp.16894-16901, 2021, 1 Dec.1. doi: 10.1109/JIOT.2021.3058587.
[4] H. Djigal, J. Xu, L. Liu and Y. Zhang, "Machine and Deep Learning for Resource Allocation in Multi-Access Edge Computing: A Survey," in IEEE Communications Surveys & Tutorials, vol. 24, no. 4, pp. 2449-2494, Fourthquarter 2022, doi: 10.1109/COMST.2022.3199544
[5] A. Shakarami, A. Shahidinejad,, & M. Ghobaei-Arani, An autonomous computation offloading strategy in Mobile Edge Computing: A deep learning-based hybrid approach. Journal of Network and Computer Applications, 178, 102974. 2021
[6] K. M. Ahmed, A. Imteaj and M. H. Amini, "Federated Deep Learning for Heterogeneous Edge Computing," 2021 20th IEEE International Conference on Machine Learning and Applications (ICMLA), Pasadena, CA, USA, pp.1146-1152, 2021. doi: 10.1109/ICMLA52953.2021.00187.
[7] A. Ndikumana, N. H. Tran, D. H. Kim, K. T. Kim and C. S. Hong, "Deep Learning Based Caching for Self-Driving Cars in Multi-Access Edge Computing," in IEEE Transactions on Intelligent Transportation Systems, May, Vol.22, No.5, pp.2862-2877, 2021. doi: 10.1109/TITS.2020.2976572.
[8] Li, J., Yin, J. & Deng, L. A robot vision navigation method using deep learning in edge computing environment. EURASIP J. Adv. Signal Process. 2021, 22 (2021). https://doi.org/10.1186/s13634-021-00734-6
[9] Y. Sun, H. Ochiai and H. Esaki, "Decentralized Deep Learning for Multi-Access Edge Computing: A Survey on Communication Efficiency and Trustworthiness," in IEEE Transactions on Artificial Intelligence, Dec., Vol.3, No.6, pp.963-972, 2022, doi: 10.1109/TAI.2021.3133819.
[10] Wang, J., Wang, M., Liu, Q. et al. Deep anomaly detection in expressway based on edge computing and deep learning. J Ambient Intell Human Comput 13, pp.1293–1305, 2022. https://doi.org/10.1007/s12652-020-02574-y
[11] S. Yang, G. Lee, & L. Huang,. Deep learning-based dynamic computation task offloading for mobile edge computing networks. Sensors, vol. 22, no. 11, 4088, 2022.
[12] D. S. Breland, S. B. Skriubakken, A. Dayal, A. Jha, P. K. Yalavarthy and L. R. Cenkeramaddi, "Deep Learning-Based Sign Language Digits Recognition From Thermal Images With Edge Computing System," in IEEE Sensors Journal, vol. 21, no. 9, pp. 10445-10453, 1 May1, 2021, doi: 10.1109/JSEN.2021.3061608.
[13] H. Sankar, V. Subramaniyaswamy, V. Vijayakumar, S. Logesh, R. Arun Kumar, & A. J. S. P Umamakeswari. Intelligent sentiment analysis approach using edge computing?based deep learning technique. Software: Practice and Experience, Vol. 50, no. 5, 645-657. 2020.
[14] K. Sundarakantham,, & E. Sivasankar. A hybrid deep learning framework for privacy preservation in edge computing. Computers & Security, Vol. 129, 103209. 2023
[15] R. Han, S. Li, X. Wang, C. H. Liu, G. Xin and L. Y. Chen, "Accelerating Gossip-Based Deep Learning in Heterogeneous Edge Computing Platforms," in IEEE Transactions on Parallel and Distributed Systems, vol. 32, no. 7, pp. 1591-1602, 1 July 2021, doi: 10.1109/TPDS.2020.3046440.
[16] B. Liu, Y. Li, , Y. Liu, Y. Guo,, & X. Chen, Pmc: A privacy-preserving deep learning model customization framework for edge computing. Proceedings of the ACM on Interactive, Mobile, Wearable and Ubiquitous Technologies, Vol. 4, No. 4, pp.1-25. 2020
[17] J. Zhu, X. Lou and W. Ye, "Lightweight Deep Learning Model in Mobile-Edge Computing for Radar-Based Human Activity Recognition," in IEEE Internet of Things Journal, vol. 8, no. 15, pp. 12350-12359, 1 Aug.1, 2021, doi: 10.1109/JIOT.2021.3063504.
[18] B. Shang, L. Liu and Z. Tian, "Deep Learning-Assisted Energy-Efficient Task Offloading in Vehicular Edge Computing Systems," in IEEE Transactions on Vehicular Technology, vol. 70, no. 9, pp. 9619-9624, Sept. 2021, doi: 10.1109/TVT.2021.3090179.
[19] Shen, T., Gao, C. & Xu, D. The analysis of intelligent real-time image recognition technology based on mobile edge computing and deep learning. J Real-Time Image Proc 18, 1157–1166 (2021). https://doi.org/10.1007/s11554-020-01039-x
[20] T. Tan and G. Cao, "Deep Learning Video Analytics Through Edge Computing and Neural Processing Units on Mobile Devices," in IEEE Transactions on Mobile Computing, vol. 22, no. 3, pp. 1433-1448, 1 March 2023, doi: 10.1109/TMC.2021.3105953.
[21] A. Gumaei et al., "Deep Learning and Blockchain with Edge Computing for 5G-Enabled Drone Identification and Flight Mode Detection," in IEEE Network, vol. 35, no. 1, pp. 94-100, January/February 2021, doi: 10.1109/MNET.011.2000204.
[22] T. Zhu, L. Kuang, J. Daniels, P. Herrero, K. Li and P. Georgiou, "IoMT-Enabled Real-Time Blood Glucose Prediction With Deep Learning and Edge Computing," in IEEE Internet of Things Journal, vol. 10, no. 5, pp. 3706-3719, 1 March 1, 2023, doi: 10.1109/JIOT.2022.3143375.
[23] Pardos, A., Menychtas, A. & Maglogiannis, I. On unifying deep learning and edge computing for human motion analysis in exergames development. Neural Comput & Applic 34, 951–967 (2022). https://doi.org/10.1007/s00521-021-06181-6
[24] M. Farooq, & M. Hassan. IoT smart homes security challenges and solution. International Journal of Security and Networks, Vol. 16. No. 4, 235-243. 2021
[25] J. Han, G. H. Lee, J. Lee, T. Y. Kim and J. K. Choi, "A Novel Deep-Learning-Based Robust Data Transmission Period Control Framework in IoT Edge Computing System," in IEEE Internet of Things Journal, Vol.9, No. 23, pp. 23486-23505, 1 Dec.1, 2022, doi: 10.1109/JIOT.2022.3203156.
[26] Wang, Z., Lv, T., & Chang, Z. Computation offloading and resource allocation based on distributed deep learning and software-defined mobile edge computing. Computer Networks, 205, 108732. 2022
[27] S. Deng, H. Zhao, W. Fang, J. Yin, S. Dustdar and A. Y. Zomaya, "Edge Intelligence: The Confluence of Edge Computing and Artificial Intelligence," in IEEE Internet of Things Journal, Vol.7, No.8, pp.7457-7469, 2020, doi: 10.1109/JIOT.2020.2984887.
[28] Hilal, A.M., Alohali, M.A., Al-Wesabi, F.N. et al. Enhancing quality of experience in mobile edge computing using deep learning based data offloading and cyberattack detection technique. Cluster Comput 26, pp.59–70, 2023. https://doi.org/10.1007/s10586-021-03401-5
[29] Kim, H.-m.; Lee, K.-h. IIoT Malware Detection Using Edge Computing and Deep Learning for Cybersecurity in Smart Factories. Appl. Sci. 12, 7679. 2022, https://doi.org/10.3390/app12157679
[30] M. Farooq, Supervised Learning Techniques for Intrusion Detection System based on Multi-layer Classification Approach. International Journal of Advanced Computer Science and Applications, vol. 13, No. 3. 2022
[31] C. S. Arvind, R. Jyothi, K. Kaushal, G. Girish, R. Saurav and G. Chetankumar, "Edge Computing Based Smart Aquaponics Monitoring System Using Deep Learning in IoT Environment," 2020 IEEE Symposium Series on Computational Intelligence (SSCI), Canberra, ACT, Australia, 2020, pp. 1485-1491, doi: 10.1109/SSCI47803.2020.9308395.
[32] Vijayasekaran, G., Duraipandian, M. An Efficient Clustering and Deep Learning Based Resource Scheduling for Edge Computing to Integrate Cloud-IoT. Wireless Pers Commun 124, 2029–2044 (2022). https://doi.org/10.1007/s11277-021-09442-8
[33] Farooq, M., & Khan, M. H. Signature-Based Intrusion Detection System in Wireless 6G IoT Networks. Journal on Internet of Things, Vol. 4, No. 3. 2022
[34] Albanese, A., Nardello, M., & Brunelli, D. Low-power deep learning edge computing platform for resource constrained lightweight compact UAVs. Sustainable Computing: Informatics and Systems, 34, 100725. 2022.
[35] P. Hao and Y. Zhang, "EDDL: A Distributed Deep Learning System for Resource-limited Edge Computing Environment," 2021 IEEE/ACM Symposium on Edge Computing (SEC), San Jose, CA, USA, pp.1-13, 2021. doi: 10.1145/3453142.3491286.
[36] Farooq, M., & Khan, M. H. Artificial Intelligence-Based Approach on Cybersecurity Challenges and Opportunities in The Internet of Things & Edge Computing Devices. International Journal of Engineering and Computer Science, Vol.12, No.7, pp.25763-25768, 2023.
[37] Lee, S. H. Real-time edge computing on multi-processes and multi-threading architectures for deep learning applications. Microprocessors and Microsystems, 92, 104554. 2022.
[38] Wei, B., Xie, Z., Liu, Y., Wen, K., Deng, F., & Zhang, P. Online monitoring method for insulator self-explosion based on edge computing and deep learning. CSEE Journal of Power and Energy Systems, Vol.8, No.6, pp.1684-1696. 2021.
[39] Zhang, D., Cao, L., Zhu, H., Zhang, T., Du, J., & Jiang, K. Task offloading method of edge computing in internet of vehicles based on deep reinforcement learning. Cluster Computing, Vol.25, No.2, pp.1175-1187. 2022.
[40] Farooq, M., Khan, R., & Khan, M. H. Stout Implementation of Firewall and Network Segmentation for Securing IoT Devices. Indian Journal of Science and Technology, Vol.16, No. 33, pp.2609-2621. 2023.
You do not have rights to view the full text article.
Please contact administration for subscription to Journal or individual article.
Mail us at ijsrnsc@gmail.com or view contact page for more details.